For years, legacy modernization has been slow, expensive, and unpredictable. But in 2025, AI is fundamentally changing the game. Enterprises are no longer limited to traditional, manual migration methods. AI-powered modernization offers a smarter, faster, and more cost-effective path.
However, not all AI solutions deliver. Copilots and accelerators promise efficiency but often fail when applied to real-world enterprise systems. They struggle with complex dependencies, inconsistent legacy code, and business-critical workflows, leaving companies with fragmented, incomplete modernization efforts.
2025 is the tipping point. The technology, expertise, and urgency have all converged:
- AI, cloud, and automation have reached enterprise maturity, making large-scale modernization feasible.
- The cost of inaction is rising; security risks, licensing fees, and maintenance costs keep climbing.
- Businesses are recognizing the need for organization-wide Gen AI integration but 32% of legacy systems can’t connect to Gen AI tools. Businesses are recognizing this, with 57% planning to modernize up to half their systems specifically to make Gen AI work for them.
- On par with that, companies are struggling to integrate AI-driven automation, real-time analytics, and cloud-native capabilities without modern infrastructures, falling behind more agile competitors.
So, how does AI truly transform modernization? What makes 2025 different? And how can enterprises leverage AI to modernize faster, smarter, and with lower risk?
In this blog, we’ll break down the shift in approach, the roadmap for AI-driven modernization, and what businesses need to know when preparing for modernization initiatives.
Let’s get into it!
Comparing the application modernization process in 2025
Traditional approaches—whether full rewrites, lift-and-shift migrations, or phased refactoring—have been plagued by long timelines, high costs, and uncertain returns.
Organizations often spend years modernizing their systems, only to encounter compatibility issues, budget overruns, or performance bottlenecks.
- High costs: Legacy system overhauls require extensive manual effort from architects, developers, and QA teams, making modernization expensive.
- Long timelines: Traditional approaches take years to execute due to complex dependency mapping, code rewrites, and iterative testing.
- Uncertain ROI: Businesses struggle to estimate modernization success upfront, leading to stalled projects or incomplete transformations.
- Fragmented execution: Most legacy-to-modern transitions involve multiple vendors and tools, creating inefficiencies and integration gaps.
- Technical debt retention: Many “modernized” applications still inherit inefficiencies from their legacy architectures, limiting long-term benefits.
Generative AI, combined with compiler technology and automated transformation frameworks, is eliminating traditional bottlenecks. Instead of relying solely on human-led code analysis, Gen AI rapidly scans and translates legacy applications with unprecedented accuracy.
- Cost efficiency: AI reduces manual intervention, cutting down labor costs and reducing total modernization expenses.
- Accelerated timelines: AI-powered code translation, dependency analysis, and automated testing compress modernization timelines from years to months.
- Predictable ROI: AI-driven analytics provide upfront estimations on cost, effort, and impact before modernization begins.
- Seamless execution: AI unifies the modernization workflow, automating everything from code conversion to optimization, reducing integration gaps.
- Optimized code quality: Unlike traditional methods that carry over technical debt, AI ensures performance-tuned, modularized, and future-ready codebases.
Traditional vs. AI-powered modernization: A side-by-side breakdown
Factor | Traditional Modernization | AI-Powered Modernization |
Time required | 1-3 years on average | 6-12 months with AI acceleration |
Cost | High labor costs due to manual effort | Reduced cost due to automation and AI-driven insights |
Dependency analysis | Requires extensive manual mapping, slowing down progress | AI rapidly scans and identifies dependencies in minutes |
Code translation | Manual rewrites or refactoring, prone to human error | AI-driven automated code conversion with higher accuracy |
Testing & debugging | Requires separate test cycles and heavy manual intervention | AI auto-generates test cases and continuously validates code |
Integration with cloud and AI | Complex, requiring additional development | AI ensures cloud-native compatibility from the start |
ROI visibility | Uncertain, often realized late | Predictable, with AI-driven forecasting models |
Risk factors | High risk of scope creep, project failures | Lower risk with AI-driven automation and real-time optimizations |
Now, let’s explore how AI-driven modernization works in practice and the step-by-step roadmap that sets it apart.
A new roadmap for AI-powered legacy modernization
Traditional modernization roadmaps are built around uncertainty – lengthy assessments, fragmented execution, and unpredictable outcomes. AI changes this dynamic by introducing automation, intelligence, and predictability at every stage.
Instead of manual code reviews and trial-and-error approaches, enterprises now have a structured, data-driven roadmap powered by AI.
Here’s how enterprises should look at application modernization roadmaps in 2025.
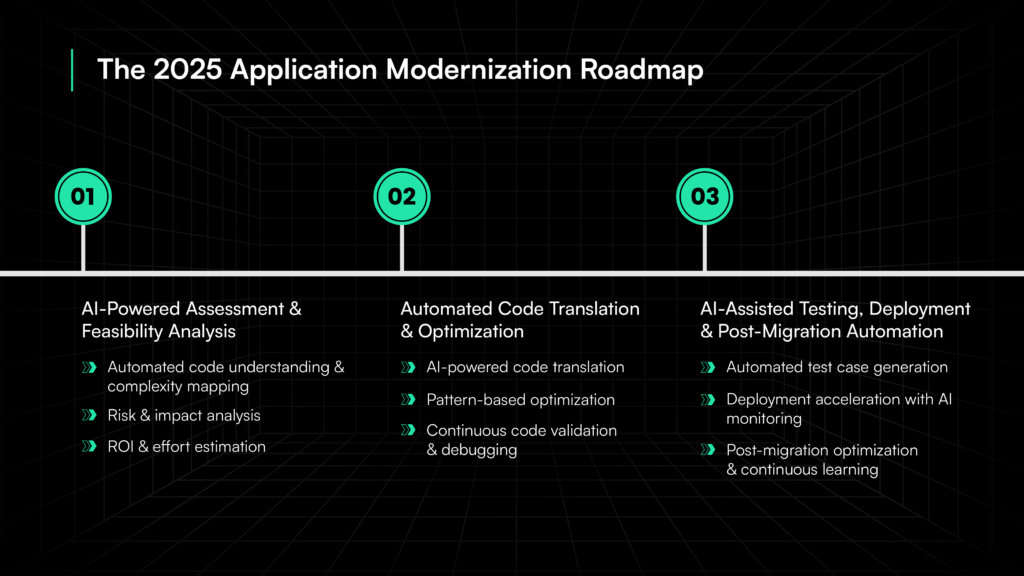
Step 1: AI-powered assessment & feasibility analysis
Before modernization begins, enterprises need clarity on complexity, risks, and expected returns. AI-driven assessment provides this clarity with unmatched speed and accuracy.
1. Automated code understanding & complexity mapping
Legacy codebases often contain years’ worth of technical debt, undocumented dependencies, and outdated logic. Traditionally, teams spend months manually analyzing these elements. AI revolutionizes this by:
- Scanning entire codebases automatically to identify dependencies, redundant code, and modernization blockers.
- Providing in-depth application comprehension and documentation for all involved stakeholders, technical or not.
- Utilizing Large Language Models (LLMs) to analyze architecture, detect legacy patterns, and assess compatibility with modern frameworks.
- Mapping out technical debt and highlighting refactoring opportunities that enhance performance and maintainability.
2. Risk & impact analysis
Modernization isn’t just about rewriting code—it’s about mitigating risks and ensuring business continuity. AI provides:
- Automated risk assessment, flagging high-risk modules that may require extra validation or refactoring.
- Phased migration strategies, identifying which components should be modernized first to minimize disruption.
- Impact reports that quantify how modernization will affect performance, security, and integration with existing systems.
3. ROI & effort estimation
One of the biggest modernization challenges has always been uncertainty around cost and effort. AI removes this uncertainty by:
- Predicting cost, time, and resource allocation based on past transformation data and real-time analysis.
- Providing side-by-side ROI comparisons between traditional and AI-driven modernization.
- Allowing leadership teams to make data-backed decisions before committing to full-scale transformation.
Step 2: Automated code translation & optimization
Once enterprises have full visibility into their legacy systems, the next challenge is execution, where they transform outdated applications into modern, maintainable architectures.
Traditionally, this phase has been slow, expensive, and error-prone. AI fundamentally changes this by automating code conversion, optimizing structure, and ensuring real-time validation.
1. AI-powered code translation
Legacy systems often rely on outdated languages like VB6, EJB, and ASP, making migration complex and resource-intensive. AI-driven code translation streamlines this process by:
- Leveraging LLMs and compiler technology to automatically convert legacy code into modern languages like C#, Java, or Python.
- Preserving core functionality while improving maintainability and scalability.
- Detecting inefficiencies in legacy logic and restructuring code to align with best practices.
2. Pattern-based optimization
Beyond translation, AI actively improves code quality by identifying and optimizing inefficient patterns. This ensures that modernized applications are not just functionally equivalent but also more efficient. AI achieves this by:
- Detecting repetitive coding patterns and refactoring them into reusable, modular components.
- Eliminating code bloat by reducing redundancy and improving maintainability.
- Suggesting architectural enhancements to align with microservices, cloud-native frameworks, or event-driven models.
3. Continuous code validation & debugging
In traditional modernization, debugging and validation introduce costly delays. AI eliminates these bottlenecks through:
- Automated syntax and logic validation, flagging errors in real time.
- AI-driven test case generation that ensures stability before deployment.
- Reducing manual debugging efforts by over 50%, freeing up developer resources for higher-value tasks.
Step 3: AI-assisted testing, deployment & post-migration automation
Once the code is modernized, the final challenge is ensuring flawless execution, deployment, and long-term optimization. Traditionally, post-migration phases introduce delays, performance issues, and maintenance overhead.
AI fundamentally transforms this process by automating testing, deployment monitoring, and continuous optimization, ensuring a seamless transition with minimal disruption.
1. Automated test case generation
Legacy modernization often breaks functionality due to inconsistencies between old and new systems. AI eliminates this risk by:
- Automatically generating test cases based on both legacy and refactored code, ensuring functional parity.
- Reducing reliance on manual test scripting, which speeds up the QA process.
- Performing AI-driven regression testing to catch unintended errors early.
2. Deployment acceleration with AI monitoring
Moving from development to production is another common bottleneck. AI streamlines deployment by:
- Detecting runtime inefficiencies and suggesting real-time performance tuning.
- Ensuring applications remain scalable and performant in cloud-native and hybrid environments.
- Minimizing downtime and rollback risks by predicting failure points before they occur.
3. Post-migration optimization & continuous learning
Modernization isn’t a one-time event—it’s an ongoing process. AI ensures sustained improvements by:
- Tracking application performance, user behavior, and error patterns post-deployment.
- Identifying and fixing recurring issues before they impact end users.
- Feeding insights back into the modernization cycle, enabling AI-driven updates and future-proofing applications.
By integrating Gen AI at every stage of the modernization process—from comprehension to deployment to long-term optimization—enterprises can ensure that modernization delivers sustained value.
Modernization no longer has to be a costly, risky endeavor. With the new Gen AI-powered playbook, it’s an automated, intelligent, and strategic evolution.
The AI-driven application modernization approach
On average, businesses spend $3M per year just to keep outdated systems running. In fact, 65% of companies now allocate over $2M annually to legacy upkeep, often just to patch inefficiencies rather than drive innovation.
So it’s clear that for years, mid-market companies have been stuck between two impossible choices: either continue maintaining outdated systems at enormous costs or embark on a modernization journey so expensive and complex that it often fails before it even begins.
Take the case of a national fuel distribution software company we recently worked with.
With a revenue of £5 million per year, they knew modernization was inevitable. But if the price tag was a million dollars upfront, it simply wasn’t feasible. However, if the cost was structured efficiently—say, $100,000 over five years—it became a viable, strategic investment.
That’s the gap we’ve filled with AI-driven modernization today. By reducing costs by 40-50%, companies that once saw modernization as out of reach can now make it part of their growth strategy.
Beyond accelerators: Why we built Legacyleap
At Legacyleap, we’ve seen firsthand how difficult legacy modernization can be. Systems built on EJB, VB6, and ColdFusion were never designed to integrate with modern architectures, making the transition incredibly challenging.
We initially experimented with accelerators, hoping they would simplify the process. But they barely moved the needle. Then, when GitHub Copilot launched in 2021, we were optimistic—it seemed like AI could finally solve legacy modernization.
But we quickly realized that off-the-shelf AI models weren’t built for the complexity of legacy systems. Copilot could generate snippets of code, but it couldn’t handle deep dependencies, tangled business logic, or architectural transformations.
That’s why we built Legacyleap—a Gen AI-powered companion platform designed specifically for application modernization. Unlike generic AI tools, we don’t stop at generating code—we assess, interpret, and validate the entire modernization process.
If you’re looking for a more detailed comparison of Legacyleap vs Copilots, check out our blog on how purpose-built modernization beats general AI tools.
Gen AI + Compiler Tech
What makes Legacyleap different is our hybrid approach. Most AI-driven modernization tools rely solely on LLMs to generate new code. But legacy systems aren’t just about code—they come with decades of dependencies, business logic, and undocumented intricacies.
Legacyleap combines Gen AI with advanced compiler technologies to:
- Understand legacy applications at a deeper level—identifying dependencies, redundant logic, and modernization blockers.
- Preserve functionality and intent while optimizing for modern architectures.
- Integrate Gen AI into every phase of modernization—from assessment to translation, testing, and deployment—without disrupting workflows.
As our founder puts it:
“We don’t just feed your code into OpenAI and hope for the best. We’ve built sophisticated layers around code understanding and representation. By combining Gen AI with compiler technologies, we ensure accuracy, reliability, and real-world applicability.
– Murali Vivekanandan, Founder”
This approach is what makes Legacyleap the only truly comprehensive Gen AI-powered modernization platform. It’s not just about automating code migration—it’s about making modernization faster, more cost-effective, and future-proof.
Risks & considerations: AI vs. traditional modernization risks
Whether AI-driven or traditional, every modernization approach comes with its own risks. The key is understanding these risks and designing a strategy that mitigates them effectively.
Traditional modernization is notorious for budget overruns, inefficiencies, and extended timelines. Manual migration efforts often stretch beyond projections due to unexpected complexities, incomplete documentation, and technical debt.
AI-driven modernization, on the other hand, significantly accelerates the process but introduces risks like AI hallucination, where models generate incorrect or suboptimal code. If left unchecked, this could lead to functionality gaps or security vulnerabilities.
Regulated industries like healthcare, finance, and government require strict data governance, compliance, and security controls. Traditional modernization methods involve human-led code reviews to ensure compliance, but they also introduce human error and inconsistencies.
With AI-powered modernization, the challenge shifts to controlling how AI interacts with enterprise data while maintaining auditability and compliance.
There are two ways in which Legacyleap does this.
Hybrid AI + Human Expertise Model
Neither a fully AI-driven nor a fully manual approach is ideal. The best approach is a hybrid model, where AI automates repetitive, high-volume tasks while human experts ensure quality, compliance, and business logic preservation.
- AI automates code migration, optimization, and validation.
- Human experts review, refine, and enforce compliance standards.
- Together, they deliver faster, more accurate, and more reliable modernization.
AI Middleware
At Legacyleap, we don’t let AI operate in isolation. Instead, we’ve designed a secure AI middleware layer that acts as a governance and validation checkpoint.
This middleware:
- Prevents AI models from directly interacting with enterprise systems to ensure security.
- Keeps sensitive data isolated while still leveraging AI for automation.
- Supports both public and private LLMs, allowing enterprises to choose the best-fit AI model while maintaining control over their data.
- Continuously validates AI-generated code to catch and correct errors before deployment.
For more info on this, check out our deep dive on The Role of AI Middleware in Gen AI-Powered Modernization.
By combining AI-driven automation with enterprise-grade security and expert oversight, Legacyleap delivers modernization that is fast, cost-effective, and risk-mitigated, ensuring that companies can modernize with confidence.
The future of modernization: AI as the new standard
By 2026, AI-driven modernization won’t be an advantage—it’ll be the expectation. The shift is inevitable, but many companies still hesitate. Change management, skills gaps, and vendor uncertainty are among the biggest blockers, leaving organizations stuck in outdated systems.
Yet, modernization isn’t just about technology, it’s about execution. Today, only 34% of companies handle app modernization fully in-house, while 31% rely entirely on external partners, and 34% take a hybrid approach.
The trend is clear: Organizations recognize that modernization requires specialized knowledge beyond internal resources. Smart modernization today means strategic collaboration, combining institutional knowledge with specialized external capabilities.
Those who wait risk falling behind in both cost efficiency and innovation, while early adopters stand to cut modernization timelines in half. See it in action with a $0 AI-powered assessment and take the first step toward an intelligent, cost-effective transformation.